Holistic AI Workshop
Assessing and Mitigating Bias and Discrimination in AI
On October 4th 2023, Roseline Polle (Responsible AI Auditor and Researcher at Holistic AI) and Sachin Beepath (AI Assurance Officer at Holistic AI) gave us a day workshop on AI Safety, focusing an assessing and mitigating bias.
Trustworthy AI
The Ethical guidelines of Trustworthy AI follows 4 main verticals:
- Robustness
- Bias
- Privacy
- Explainability
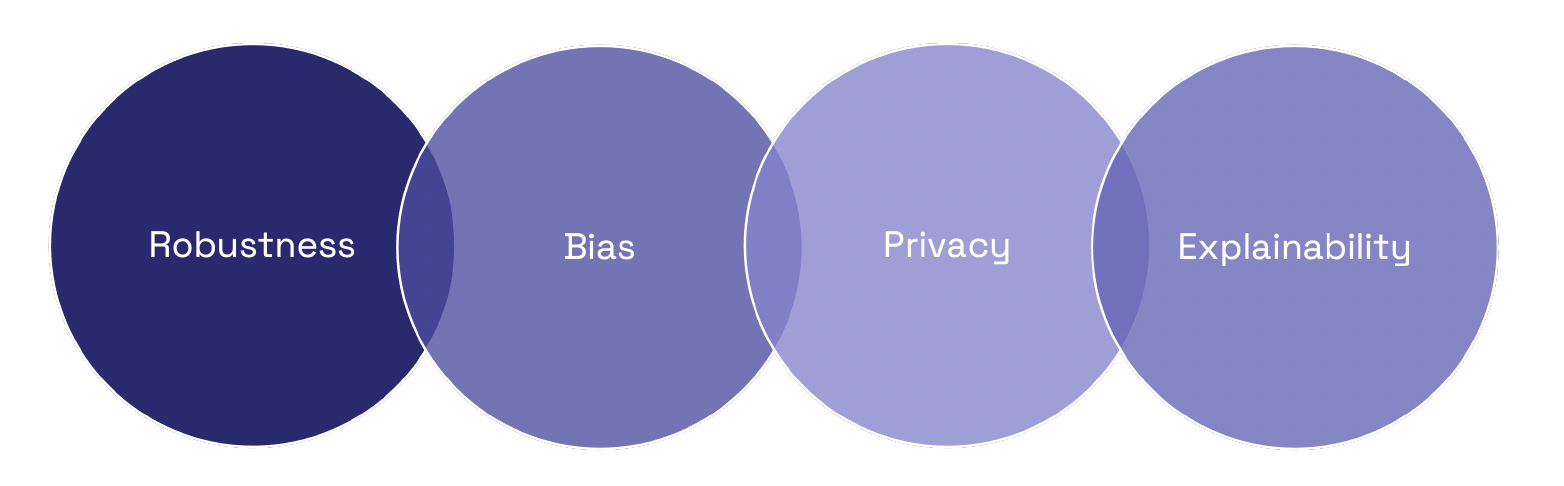
Source: Holistic AI
Case Studies
During the workshop we saw case studies for all four verticals.
- Bias:
- UK A-level grading fiasco: Grades for A-level students during the pandemic were determined using an algorithm. Unfortunately, this algorithm was subject to several sources of bias, which lead to lower-than-expected grades, protests and eventually the government retracted the grades.
- COMPAS predicted the likelihood of recidivism of criminals, but was found to have sever bias against black defendants: they were often considered to be at higher risk of recidivism, whereas white defendants were considered less risky. This was also true when controlling for prior crimes, age and gender.
- Amazon Recruitment Algorithm was found to discriminate against female candidates.
- Gender Shades algorithms from Microsoft, IBM and Face++ all perform better on lighter subjects, with a difference of up to 34% in error rates. Most of the misgendered faces by Face++ were of female subjects. Bias against intersectionality is exacerbated.
- Explainability
- Robustness
- Privacy
Sources of Bias
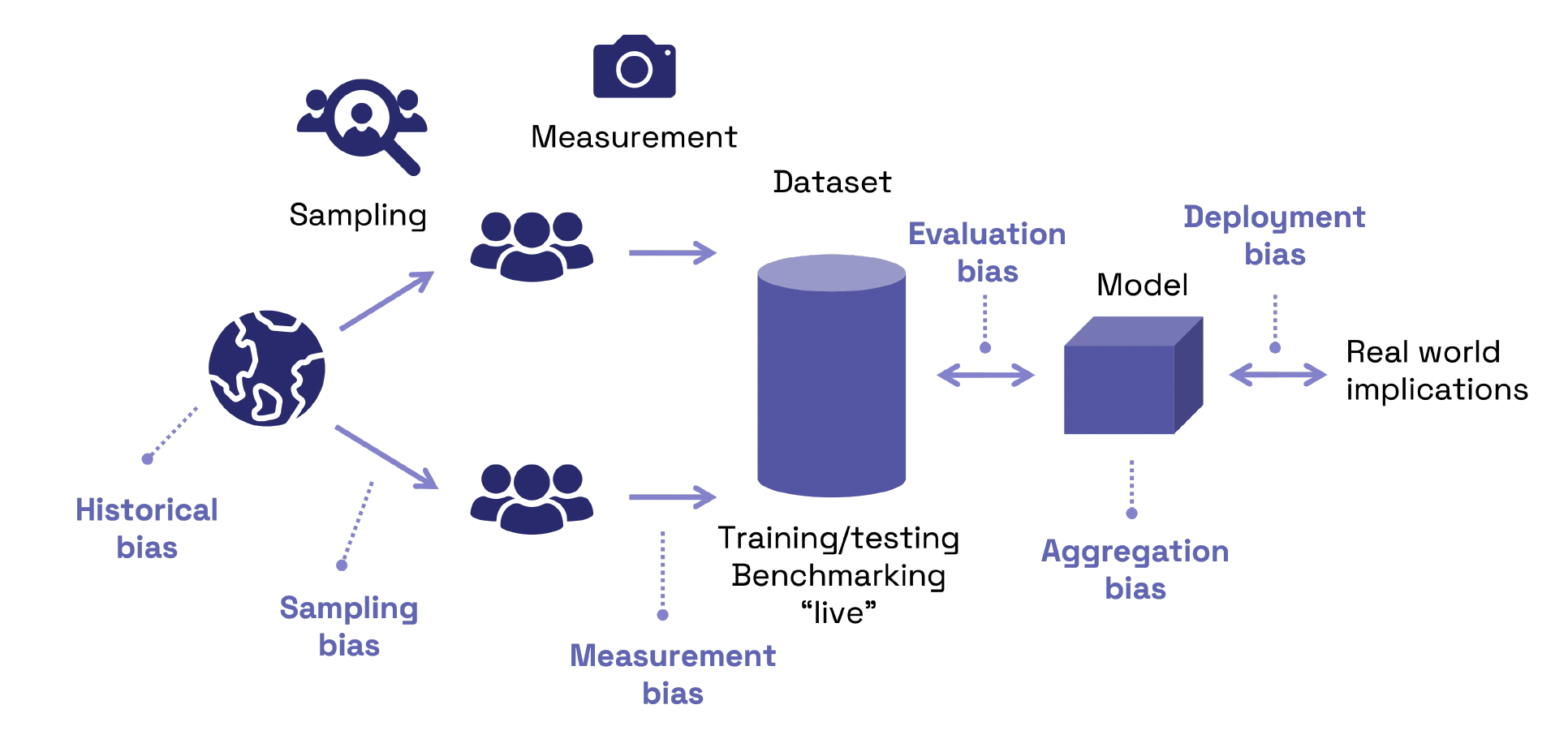
Source: Holistic AI
structured as follows:
- Teaching Session:
- 30m Talk: Introduction to Trustworthy AI, with a focus on Bias and Fairness although they touched upon other verticals such as Explainability, Privacy, and Robustness.
- 30min Talk: Sources of Bias. Equality of Opportunity vs Equality of Outcome.
- 20min Talk: Measuring Bias in Binary Classification.
- 30min Talk: Mitigating bias in Binary Classification.
- 30min Talk: Trade-offs and other verticals such as Explainability and Robustness.
- Practical Session:
- Measure and Mitigate in Binary classification using recruitment data and holisticai library (Equality of Outcome). Several mitigation techniques were explored.
- Measure and Mitigate bias in a face recognition application (Equality of Opportunity). Several mitigation techniques were explored.
- Explore trade-offs with other verticals, with a focus on explainability (SHAP, LIME, Feature importance)